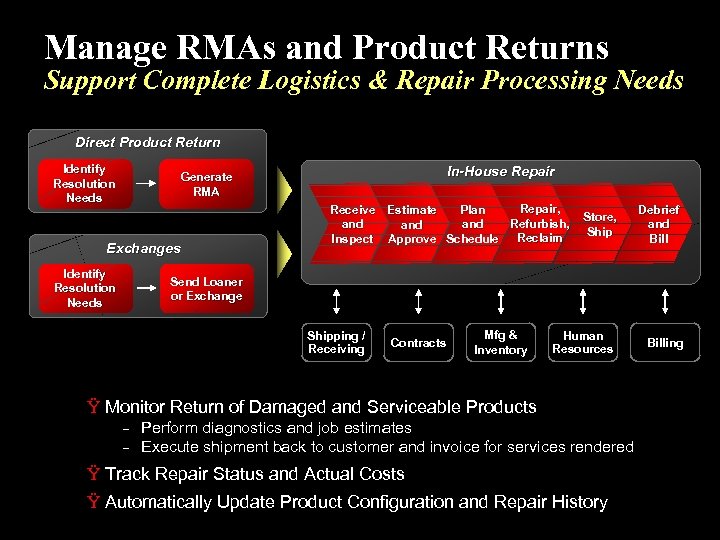
Abstract. Predictions of stock and foreign exchange (Forex) have always been a hot and profitable area of study. Deep learning applications have been proven to yield better accuracy and return in the field of financial prediction and blogger.com by: 10 /11/2 · Nassirtoussi et al. proposed an approach for forex prediction wherein the major focus was on strengthening text-mining aspects that had not been focused upon in previous studies. Dimensionality reduction, semantic integration, and sentiment analysis enabled efficient blogger.com by: 6 /6/8 · We mentioned predictions, important statements, analysis and forecasts by famous experts about cryptocurrencies. Biggest Cryptocurrency Predictions in Jed McCaleb, CTO Stellar
NLP Applications: 5 Use Cases From Text Mining to Sentiment Analysis
Financial Innovation volume 6Article number: 39 Cite this article. Metrics details. Text-mining technologies have substantially affected financial industries. As the data in every sector of finance have grown immensely, text mining has emerged as an important field of research in the domain of finance. Therefore, reviewing the recent literature on text-mining applications in finance can be useful for identifying areas for further research.
This paper focuses on the text-mining literature related to financial forecasting, banking, and corporate finance.
It also analyses the existing literature on text mining in financial applications and provides a summary of some recent studies. Finally, the paper briefly discusses various text-mining methods being applied in the financial domain, the challenges faced in text mining of new-deadlines for forex market predictions applications, and the future scope of text mining in finance. Nearly every activity in modern life, from phone calls to satellites sent into space, text mining of new-deadlines for forex market predictions, has evolved exponentially with technology Patel et al.
The increasing ability to create and manage information has been an influential factor in the development of technology. According to the National Security Agency of the United States, petabytes on average are handled daily over the Internet Hariri et al.
With the rapid increase in data and information communicated over the Internet, it has become necessary to regulate and ease the flow of the same Ahir et al. A number of commercial and social applications have been introduced for these purposes. Aspects of data and information, such as security, research, and sentiment analysis, can be of great help to organisations, governments, and the public Jani et al. There are various optimized techniques that aid us in tasks such as classification, summarisation, and ease of access and management of data, among others Shah et al.
Algorithms related to machine learning and deep learning DL are just some of the many algorithms that can be used to process the available information Kakkad et al. Even though there is a massive amount of available information, the use of computational techniques can help us process information from top to bottom and analyse entire documents as well as individual words Pandya et al. This has led to a rise in interest in methods and tools that can help extract useful information automatically from enormous amounts of unstructured data Jaseena and David ; David and Balakrishnan One crucial method is text mining, which is a combined derivative of techniques such as data mining, machine learning, and computational linguistics, among others.
Text mining aims to extract information and patterns from textual data Talib et al. The trivial approach to text mining is manual, text mining of new-deadlines for forex market predictions, in which a human reads the text and searches for useful information in text mining of new-deadlines for forex market predictions. A more logical approach is automatic, which mines text in an efficient way in terms of speed and cost Herranz et al.
This value only provides us with a limited indication of the actual size and reach of the global finance industry. Technology has paved the way for digitalisation in this rapidly growing behemoth. Marrara et al. FinTech has popularised the use of data in the financial industry.
This data is substantially in the form of structured or unstructured text. Therefore, traditionally and technically, textual data can be regarded as always having been a prevailing and essential element in the finance sector.
Unstructured textual data have been increasing rapidly in the finance industry Lewis and Young This is where text mining has a lot of potential. Kumar and Ravi explored various applications in the financial domain in which text mining could play a significant role. They concluded that it had numerous applications in this industry, such as various kinds of predictions, customer relationship management, and cybersecurity issues, among others.
Many novel methods have been proposed for analysing financial results in recent years, and artificial intelligence has made it possible to analyse and even predict financial outcomes based on historical data.
Finance has been an important force in human life since the earliest civilisations. It is noteworthy that from barter systems to cryptocurrencies, finance has always been associated with data, such as transactions, accounts, prices, and reports. Manual approaches to processing data have been reduced in use and significance over time. Researchers and practitioners have come to prefer digitised and automated approaches for studying and analysing financial data.
Financial data contain a significant amount of latent information. If the latent information were to be extracted manually from a huge corpus of data, it might take years. Advancements in text mining have made it possible to efficiently examine textual data pertaining to finance. Bach et al. They structured the review in terms of three critical questions. These questions pertained to the intellectual core of finance, the text-mining techniques used in finance, and the data sources of financial sectors.
Kumar and Ravi discussed the model presented by Vu et al. They also mentioned the model of Lavrenko et al. We will further discuss text-mining applications in finance in subsequent sections. Apart from finance, we present a brief overview of text mining in other industries. On social media, people generate text data in the form of posts, blogs, and web forum activity, among many others Agichtein et al.
Despite the vast quantity of data available, the relatively low proportion of content of significant text mining of new-deadlines for forex market predictions is still a problem Kinsella et al.
In the biomedical field too, there is a need for effective text-mining and classification methods Krallinger et al. On e-commerce websites, text mining is used to prevent the repetition of information to the same audience Da-sheng et al, text mining of new-deadlines for forex market predictions. In healthcare, researchers have worked on applications such as the identification of healthcare topics directly from personal messages over the Internet Luclassification of online data Srivastava et al.
The agriculture industry has also used text mining in, for example, the classification of agricultural regulations Espejo-Garcia et al.
Text mining has also been utilised in the detection of malicious web URLs which evolve over time and have complex features Li et al. This paper discusses the use of text mining in the financial domain in detail, taking into consideration three major areas of application: financial forecasting, banking, and corporate finance.
We also discuss the widely used methodologies and techniques for text mining in finance, the challenges faced by researchers, and the future scope for text-mining methods in finance. Text mining is a process through which the user derives high-quality information from a given piece of text. Text mining has seen a significant increase in demand over the last few years.
Coupled with big data analytics, the field of text mining is evolving continuously. Finance is one major sector that can benefit from these techniques; the analysis of large volumes of financial data is both a need and an advantage for corporates, government, and the general public.
This section discusses some important and widely used techniques in the analysis of textual data in the context of finance. One of the most important techniques in the field is SA. It has applications in numerous sectors. This technique extracts the underlying opinions within textual data and is therefore also referred to as opinion mining Akaichi et al.
It is of prime use in a number of domains, such as e-commerce platforms, blogs, online social media, and microblogs. The motives behind sentiment analysis can be broadly divided into emotion recognition and polarity detection. Emotion detection is focused on the extraction of a set of emotion labels, and polarity detection is more of a classifier-oriented approach with discrete outputs e. There are two main approaches for SA, namely lexicon-based dictionary-based and machine learning ML.
The latter is text mining of new-deadlines for forex market predictions classified into supervised and unsupervised learning approaches Xu et al. Lexicon-based approaches use SentiWordNet word maps, whereas ML considers SA as a classification problem and uses established techniques for it.
In lexicon-based approaches, the overall score for sentiment is calculated by dividing the sentiment frequency by the sum of positive and negative sentiments, text mining of new-deadlines for forex market predictions.
In ML approaches, the major techniques that are used are Naïve Bayes NB classifier and support vector machines SVMswhich use labelled data for classification. However, ML requires domain-specific datasets, which can be considered as a limitation Al-Natour and Turetken After data preprocessing, feature selection is performed as per the requirement, following which one obtains the final results after the analysis of the given data as per the adopted approach Hassonah et al.
In the financial domain, stock market prediction text mining of new-deadlines for forex market predictions one of the applications in which SA has been used to predict future stock market trends and prices from the analysis of financial news articles. Joshi et al. Renault used StockTwits a platform where people share ideas about the stock market as a data source and applied five algorithms, namely NB, a maximum entropy method, text mining of new-deadlines for forex market predictions, a linear SVM, an RF, and a multilayer perceptron and concluded that the maximum entropy and linear SVM methods gave the best results.
Over the years, researchers have combined deep learning methods with traditional machine learning techniques e. Information extraction IE is used to extract predefined data types from a text document. IE systems mainly aim for object identification by extracting relevant information from the fragments and then putting all the extracted pieces in a framework.
Post extraction, DiscoTEX Discovery from TextEXtraction is one of the core methods used to convert the structured data into meaningful data to discover knowledge from it Salloum et al. In finance, named-entity recognition NER is used for extracting predefined types of data from a document. In banking, transaction order documents of customers may come via fax, text mining of new-deadlines for forex market predictions, which results in very diverse documents because of the lack of a fixed template and creates the need for proper feature extraction to obtain a structured document Emekligil et al.
NLP is a part of the artificial intelligence domain and attempts to help transform imprecise and ambiguous messages into unambiguous and precise messages. It is often used to mine documents to obtain insights for developing conclusions Fisher et al. NLP can help perform various analyses, such as NER, which further helps in identifying the relationships and other information to identify the key concept.
However, NLP lacks a dictionary list for all the named entities used for identification Talib et al. As NLP is a pragmatic research approach to analyse the huge amount of available data, Xing et al. Figure 1 provides an intuitive grasp of natural language-based financial forecasting NLFF. An intersection of NLP and financial forecasting to illustrate the concept of NLFF Xing et text mining of new-deadlines for forex market predictions. Chen et al.
They reviewed three aspects, namely know your customer KYCtext mining of new-deadlines for forex market predictions, know your product KYPand satisfy your customer SYC.
In KYC, a lot of textual data is generated in the process text mining of new-deadlines for forex market predictions acquiring information about customers corporate sector and retail. With respect to KYP, salespersons are required to know all the attributes of their product, which again requires data in order to know the prospects, risks, and opportunities of the product.
Herranz et al. Text classification is a four-step process comprising feature extraction, dimension reduction, classifier selection, and evaluation. Feature extraction can be done with common techniques such as term frequency and Word2Vec; then, dimensionality reduction is performed using techniques such as principal component analysis text mining of new-deadlines for forex market predictions linear discriminant analysis. Choosing a classifier is an important step, and it has been observed that deep learning approaches have surpassed the results of other machine learning algorithms.
The evaluation step helps in understanding the performance of the model; it is conducting using various parameters, such as the Matthews correlation coefficient MCCarea under the ROC curve AUCand accuracy.
#1 Weekly Forex Forecast 15th-19th Mar [Learn How To Trade Correctly]
, time: 19:2210 Biggest Cryptocurrency Predictions in - Insider Monkey

Abstract. Predictions of stock and foreign exchange (Forex) have always been a hot and profitable area of study. Deep learning applications have been proven to yield better accuracy and return in the field of financial prediction and blogger.com by: 10 /4/22 · Nassirtoussi AK, Aghabozorgi S, Wah T-Y, Ngo DCL () Text-mining of news-headlines for FOREX market prediction: a multi-layer dimension reduction algorithm with semantics and sentiment. Expert Syst Appl – Article Google Scholar27 /1/1 · FOREX prediction through text mining of news is viable and effective. • Feature-selection by abstraction of word-hypernyms increases prediction accuracy. • Feature-weighting based on the sum of pos and neg sentiment scores is effective. • Feature-reductionCited by:
No comments:
Post a Comment